3 Critical Steps to Optimize Machine Learning for Clinical Development
Why “Resistance to Change” Is the Biggest Blocker to Clinical Development Innovation
We don’t just mean improved clinical outcomes but also better feasibility, PI and site selection, patient recruitment, and diversity outcomes. However, when the conversation turns to machine learning (ML), “resistance to change” among potential users is often the primary blocker to adoption.
Nonetheless, embracing innovation, including ML, with the ever-increasing volume, velocity, and variety of clinical data is essential to improving clinical outcomes.
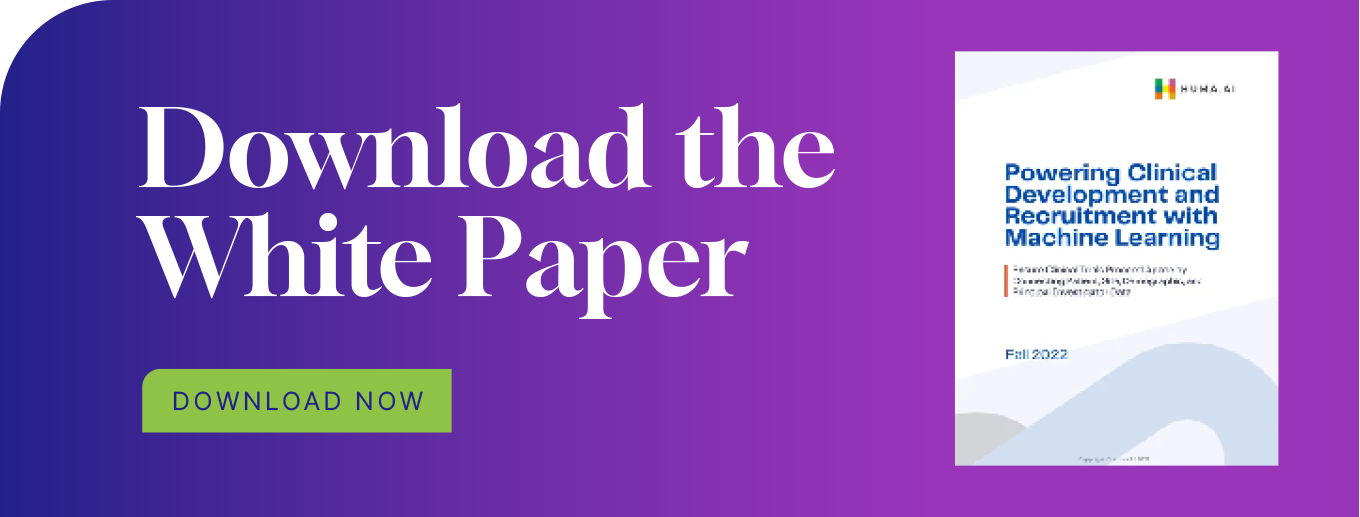
Therefore, data scientists and clinical development professionals must work together to communicate the benefits of an innovation upfront. Additionally, the innovation must function correctly and present a great user experience to be successful. By meeting these fundamental requirements, a clinical development innovation can be impactful, relevant, and easy to use.
According to McKinsey: “The clinical environment is changing rapidly and simultaneously becoming more complex: the rise of personalized medicine has led to increasingly complex protocols; trials today are more often targeted at smaller patient populations that are also harder to find, while competition for sites and patients is becoming ever more fierce.”
Specifically, to overcome resistance to change, be sure to follow these three steps.
1. Deliver Benefits that Are Clear and Quantifiable
In other words, tell people why they should care.
For any new technology to be successfully adopted, the first step is to ensure the benefits are clear and quantifiable. That means if users can see how your innovation will make their lives easier, they are more likely to be willing to try it out.
For example, suppose a data-driven innovation promises to speed up clinical trials by 10% but cannot provide hard data to back up this claim. In that case, users will likely be hesitant to invest time and resources into learning how to use the new tool.
On the other hand, if an ML-based solution can provide concrete evidence that it will improve patient outcomes or reduce costs associated with clinical trials, clinical development professionals will be much more likely to take notice.
For data scientists and clinical development professionals to work together effectively, they must communicate openly about the benefits each stakeholder group expects to gain from adopting new technologies.
By clearly outlining the benefits of an ML-based innovation upfront, data scientists can help clinical development professionals understand how the new tool can improve existing workflows.
2. Function Seamlessly (the First Time)
The first time users try a new ML solution, it must work.
Nothing repels users faster than glitches or errors the first time they try something new. Already wary of change, many users are looking for reasons not to trust ML-based solutions. So if they immediately encounter problems, many are likely to give up on it entirely.
Therefore, the second step is getting it right the first time.
Ensuring that innovation functions properly requires time and effort to test and debug it properly. This process can be long and tedious, but it is essential to ensuring that innovation is successful. Once all the bugs have been resolved, users will have a more positive experience that reinforces future use.
Without a doubt, there will be times when users encounter unexpected problems or glitches while using an innovation.
These issues might seem minor initially, but they can quickly derail user experience and cause clinical development professionals to give up on that innovation altogether. To prevent this from happening, vigilance and continuous monitoring of the innovation performance are needed. Users should offer feedback and analyze data to identify potential problems or improvement areas. With careful attention and dedication, you can create a fully functional and reliable innovation that will satisfy even the most demanding clinical development professionals.
In the end, however, the “behind the scenes” effort required to impress users always pays off.
3. Provide a Great User Experience (UX)
Understand what users want and adapt to their changing needs.
The third step for any ML-based clinical development innovation is the ability to provide a quality user experience.
This involves incorporating intuitive design and functionality that is simple and easy to use while providing a level of customization and personalization to ensure that users feel fully supported throughout their clinical development journey. At the same time, it is essential to build trust with clinical development professionals by demonstrating the efficacy of your innovative approach.
This can be achieved through thorough testing, data analysis, and validation from established subject matter experts and leaders.
Another key consideration is ensuring that your solution adapts to changing needs over time.
Whether driven by advances in technology or new regulatory requirements, you must have the flexibility to continually evolve your ML-based innovation to remain relevant, impactful, and effective at addressing users’ unique needs across different stages of clinical development.
If you can meet these crucial requirements for clinical development innovations based on machine learning, you will pave the way for widespread adoption and success in this rapidly evolving field.
In Summary
Following these three tips can increase the chances that clinical development professionals will adopt your ML-based innovation.
As Deloitte wrote: “Value is expected to come from human-enabled AI— expert clinical knowledge coupled with AI and machine learning (ML) analysis.”
First, it is essential to communicate the benefits of your innovation upfront. Second, your innovation must function properly. Finally, your innovation should provide a great user experience.
By meeting these requirements, you will be well on your way to success in ML for clinical development.
Want Deeper Clinical Trials Insights?
Finding the sites and principal investigators to conduct trials is no small task.
With Huma.AI , however, a single intelligence layer is placed over a wide variety of data sets pulled from almost any source.
Huma.AI uncovers deeply buried clinical trial insights that give clinical professionals the highly targeted intelligence and market advantage needed to create more successful trials.